How Can Big Data Predict Natural Disasters and Reduce Risks
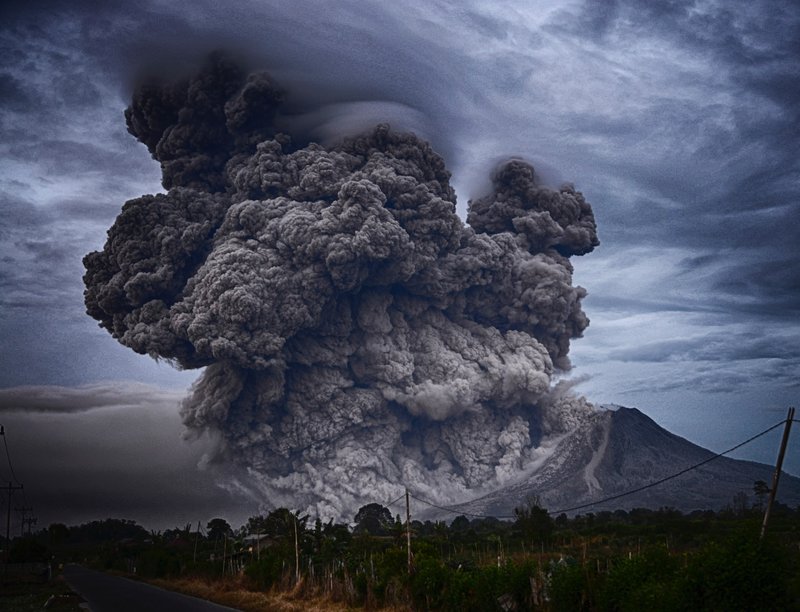
The application of big data in disaster management has gained significant attention in recent years. This article examines the potential of big data analytics in predicting natural disasters and reducing associated risks.
By analyzing historical data, leveraging real-time sensor data, and utilizing machine learning algorithms, big data can provide early warning systems for disaster preparedness.
Furthermore, the use of big data analytics enables the assessment of risks and implementation of mitigation strategies.
Collaborative efforts in this field are also discussed, highlighting the importance of collective action in disaster response and recovery.
Key Takeaways
- Big data enhances forecasting, planning, and response strategies for natural disasters.
- Analyzing historical data enables the development of early warning systems and prompt identification of hazards and vulnerabilities.
- Data-driven risk assessment helps in informed decision making, resource allocation, and identifying areas prone to disasters.
- Predictive modeling using big data can reduce loss of life and injuries, minimize property damage, and optimize resource allocation in natural disaster risk assessment.
The Role of Big Data in Disaster Preparedness
The role of big data in disaster preparedness involves the utilization of large-scale data analysis to enhance forecasting, planning, and response strategies. Big data enables data driven decision making by providing valuable insights into the patterns and trends associated with natural disasters.
By analyzing vast amounts of data collected from various sources such as weather sensors, satellite imagery, social media feeds, and historical records, researchers and emergency managers can identify potential risks and predict the occurrence of natural disasters with greater accuracy.
Moreover, big data allows for the development of data visualization techniques that enable stakeholders to interpret complex information in a more accessible manner. By visualizing data through maps, graphs, and other visual representations, decision makers can better understand the potential impacts of disasters and allocate resources effectively to mitigate risks.
Analyzing Historical Data for Early Warning Systems
This discussion focuses on the utilization of data-driven risk assessment, early detection algorithms, and predictive modeling techniques in analyzing historical data for the development of effective early warning systems.
By analyzing large volumes of historical data, organizations can identify patterns and trends that provide valuable insights into potential risks and hazards.
Early detection algorithms can then be employed to continuously monitor incoming data in real-time, enabling prompt identification of emerging threats.
Data-Driven Risk Assessment
Data-driven risk assessment in the context of natural disasters focuses on utilizing big data to evaluate the potential hazards and vulnerabilities associated with specific geographical areas. By analyzing large datasets from various sources, such as satellite imagery, weather reports, and historical records, decision-makers can make informed choices to improve disaster response. This approach allows for data-driven decision making, enabling authorities to allocate resources efficiently and effectively. For example, by identifying areas prone to flooding based on historical data, emergency services can preposition supplies and personnel to ensure a swift response. Additionally, the use of big data can aid in identifying vulnerable populations, enabling targeted evacuation plans and resource allocation. The table below demonstrates the potential benefits of data-driven risk assessment in improving disaster response:
Benefits of Data-Driven Risk Assessment |
---|
Enhanced understanding of hazards and vulnerabilities |
Improved resource allocation |
Targeted evacuation and response plans |
Early Detection Algorithms
Early detection algorithms play a crucial role in identifying potential hazards and vulnerabilities associated with specific geographical areas. These algorithms are an essential component of early warning systems, enabling timely and effective disaster response. By analyzing various data sources, such as weather patterns, seismic activities, and social media feeds, these algorithms can detect and predict the occurrence of natural disasters.
This information helps authorities and emergency services to prepare and respond proactively, mitigating the impact of disasters on human lives and infrastructure. The use of early detection algorithms in disaster response has several benefits, including:
- Enhanced preparedness and response capabilities
- Reduced loss of life and injuries
- Minimized damage to infrastructure and property
- Improved coordination among different response agencies
- Increased public awareness and participation in disaster preparedness efforts
Overall, early detection algorithms are a powerful tool in predicting and minimizing the risks associated with natural disasters, contributing to more effective disaster response strategies.
Predictive Modeling Techniques
Predictive modeling techniques involve the use of statistical algorithms and machine learning algorithms to analyze historical data and make predictions about future events or outcomes. These techniques have numerous applications in various fields, including advanced data analytics.
By analyzing large volumes of data, predictive models can identify patterns and relationships that may not be apparent to humans. This enables organizations to make more informed decisions and optimize their operations.
In the context of predictive modeling applications, advanced data analytics plays a crucial role in preprocessing and transforming raw data into a suitable format for modeling. Additionally, it helps in feature selection and engineering, which are essential for building accurate and robust predictive models.
Overall, predictive modeling techniques and advanced data analytics have revolutionized the way organizations approach decision-making and planning for future events.
Harnessing Sensor Data for Real-time Disaster Monitoring
One approach to improve real-time disaster monitoring involves utilizing sensor data to capture and analyze relevant information. This method allows for the timely detection and prediction of natural disasters, enabling proactive measures to be taken to reduce risks and mitigate potential damage. Real-time sensor data analysis provides valuable insights into various parameters such as temperature, humidity, air pressure, and seismic activity, which can help identify patterns and trends indicative of impending disasters.
Additionally, the integration of satellite data enhances the accuracy and coverage of the monitoring system, enabling a comprehensive view of the affected areas. By harnessing sensor data and leveraging advanced data analysis techniques, decision-makers can make informed decisions and implement effective strategies to minimize the impact of natural disasters on human lives and infrastructure.
- Early warning systems based on real-time data
- Enhanced situational awareness for disaster response teams
- Improved evacuation planning and coordination
- Rapid deployment of resources to affected areas
- Enhanced understanding of disaster patterns for long-term risk management
Utilizing Machine Learning to Predict Natural Disasters
Utilizing machine learning techniques allows for the analysis of historical and real-time sensor data, enabling the identification of patterns and trends indicative of potential catastrophic events. By employing machine learning algorithms, researchers and organizations can develop predictive models that aim to forecast natural disasters with greater accuracy.
These models leverage vast amounts of data from various sources, such as weather sensors, satellite imagery, and seismic readings, to generate insights and predictions. The application of machine learning in disaster prediction facilitates data-driven decision making, enabling authorities to take proactive measures to mitigate the impact of natural disasters.
Big Data Analytics for Risk Assessment and Mitigation
Predictive modeling for risks and real-time data analysis are two key components of big data analytics for risk assessment and mitigation.
Predictive modeling involves using historical data and statistical techniques to forecast future outcomes and identify potential risks.
Real-time data analysis, on the other hand, involves the continuous monitoring and analysis of data as it is generated, allowing for timely identification and response to emerging risks.
These approaches offer valuable insights and tools for organizations to proactively manage and mitigate risks in various domains.
Predictive Modeling for Risks
Risk assessment and mitigation can be improved through the application of predictive modeling techniques in the context of natural disasters. Predictive analytics and risk modeling play a crucial role in understanding and anticipating the potential impacts of natural disasters, enabling policymakers and emergency responders to take proactive measures to reduce risks.
By leveraging historical data, satellite imagery, weather patterns, and other relevant information, predictive modeling can help identify vulnerable areas, estimate the likelihood and severity of future disasters, and determine the most effective strategies for mitigating their impacts. This approach enhances decision-making processes, allowing for better resource allocation and planning, ultimately leading to more efficient and effective disaster preparedness and response efforts.
Some of the specific benefits of predictive modeling in natural disaster risk assessment and mitigation include:
- Reduced loss of life and injuries
- Minimized property damage
- Improved early warning systems
- Enhanced evacuation planning
- Optimized resource allocation
Real-Time Data Analysis
Real-time data analysis allows for the continuous monitoring and evaluation of various indicators, enabling timely identification of emerging patterns and trends in order to inform decision-making processes.
Real-time data visualization plays a crucial role in this process by providing a visual representation of the data, allowing decision-makers to quickly identify patterns and trends. This visualization can be achieved through the use of various methods, such as interactive dashboards or real-time data streaming.
Additionally, predictive analytics algorithms can be applied to real-time data analysis to forecast future events and outcomes. These algorithms use historical data and statistical models to make predictions based on current data.
Collaborative Efforts in Big Data for Disaster Response and Recovery
Collaborative efforts in utilizing big data for disaster response and recovery involve the coordination of various stakeholders and the integration of diverse datasets to enhance decision-making and facilitate effective disaster management. These collaborative partnerships and data sharing initiatives play a crucial role in improving the overall response and recovery process.
The following unordered bullet list highlights the potential benefits and emotions evoked by these collaborative efforts:
- Increased speed and efficiency in disaster response and recovery operations, leading to lives saved and reduced suffering.
- Enhanced situational awareness, allowing for better allocation of resources and targeted response efforts.
- Improved prediction and forecasting capabilities, empowering communities to better prepare for and mitigate the impact of natural disasters.
- Enhanced understanding of disaster patterns and trends, leading to more effective long-term planning and risk reduction strategies.
- Greater inclusivity and equity in disaster response and recovery, ensuring that vulnerable populations receive the support they need.
These collaborative initiatives provide a sense of hope and optimism, as they harness the power of big data to improve disaster management and build more resilient communities.
Frequently Asked Questions
How Can Big Data Help in Disaster Preparedness and What Specific Role Does It Play?
The role of technology, specifically big data, in disaster preparedness involves data-driven decision making. By analyzing large amounts of data, it can help identify patterns, trends, and potential risks, enabling proactive measures to reduce the impact of natural disasters.
What Are the Key Factors Involved in Analyzing Historical Data for Early Warning Systems?
Key factors in analyzing historical data for early warning systems include data quality, relevance, and accessibility. Effective data analysis techniques, such as statistical modeling and machine learning, can help identify patterns and trends that contribute to accurate predictions and risk reduction.
How Does the Harnessing of Sensor Data Contribute to Real-Time Disaster Monitoring?
Real-time monitoring through the analysis of sensor data contributes to the effectiveness of disaster monitoring systems. By harnessing sensor data, potential risks can be identified promptly, enabling timely actions to mitigate and reduce the impact of natural disasters.
Can You Explain the Process of Utilizing Machine Learning to Predict Natural Disasters?
The utilization of machine learning in the process of predicting natural disasters involves the application of predictive analytics techniques to analyze and interpret large volumes of data, enabling the identification of patterns and trends that may indicate the occurrence of future natural disasters.
What Are the Main Benefits of Using Big Data Analytics for Risk Assessment and Mitigation in Natural Disasters?
Utilizing big data analytics for risk assessment and mitigation in natural disasters offers several benefits. These include improved forecasting accuracy, real-time monitoring, enhanced situational awareness, and more effective resource allocation.