Can Big Data Predict Economic Trends and Market Shifts
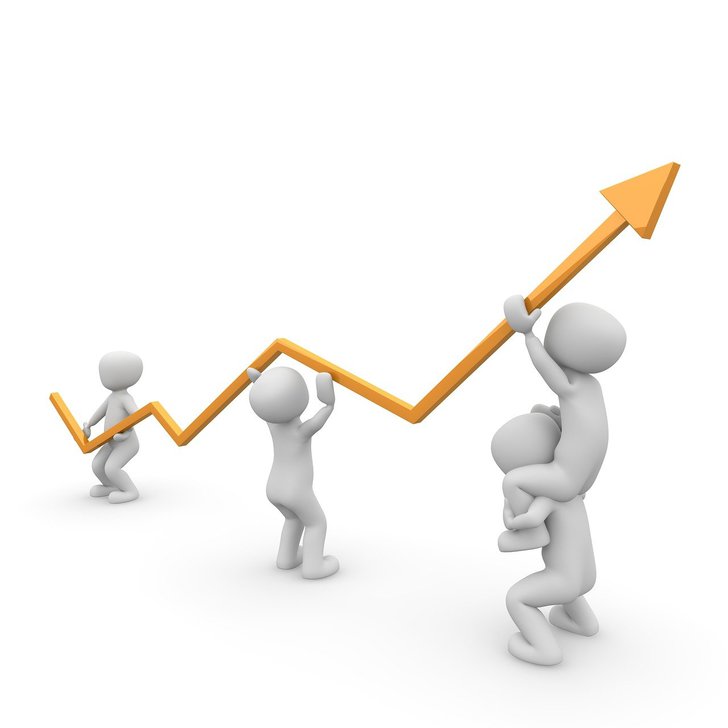
This article explores the potential of big data in predicting economic trends and market shifts.
It examines the role of big data in economic trend analysis and highlights the significance of analyzing market shifts through the lens of large-scale data analysis.
Furthermore, it discusses key data sources used for predicting economic trends and the application of machine learning techniques in economic forecasting.
The article also addresses the challenges and limitations associated with using big data for predicting market shifts, along with case studies showcasing successful applications.
Finally, it raises ethical considerations relevant to the use of big data in economic analysis.
Key Takeaways
- Big data provides new possibilities for understanding and predicting economic trends.
- Analyzing large volumes of data from various sources provides insights into consumer behavior and market trends.
- Data visualization techniques and predictive analytics identify patterns and trends in market shifts.
- Real-time data availability allows for timely analysis and decision-making in predicting economic trends.
The Role of Big Data in Economic Trend Analysis
The role of big data in economic trend analysis is a topic of interest and research in the field of economics. The availability of large and diverse datasets has opened up new possibilities for understanding and predicting economic trends.
Data analytics techniques, such as predictive modeling, play a crucial role in leveraging big data for economic analysis. These techniques involve using statistical algorithms and machine learning to analyze historical data and identify patterns and relationships that can be used to make predictions about future economic trends.
By analyzing large volumes of data from various sources, including social media, financial transactions, and government reports, economists can gain valuable insights into consumer behavior, market trends, and overall economic performance.
This enables policymakers and businesses to make informed decisions and develop strategies to adapt to changing economic conditions.
Analyzing Market Shifts Through Big Data
An analysis of information derived from large-scale datasets can provide insights into the dynamics of market changes. This analysis involves the use of data visualization techniques and predictive analytics to identify patterns and trends in the data.
By visualizing the data in a clear and concise manner, analysts can gain a better understanding of market shifts and make informed decisions.
Predictive analytics, on the other hand, uses statistical models and algorithms to forecast future market trends based on historical data. This allows businesses to anticipate market changes and adjust their strategies accordingly.
Overall, the analysis of big data plays a crucial role in understanding market shifts and can provide valuable insights for businesses to adapt and thrive in a rapidly changing economic landscape.
Key Data Sources for Predicting Economic Trends
Data accuracy and reliability, as well as real-time data availability, play crucial roles in predicting economic trends. In order to make accurate predictions, it is essential to have access to high-quality data that is free from errors or biases.
Additionally, real-time data availability allows for timely analysis and decision-making, enabling researchers to capture the most up-to-date information and trends.
Data Accuracy and Reliability
Achieving high levels of accuracy and reliability in the data used for predicting economic trends and market shifts is crucial for ensuring the validity of the results. In the context of big data analysis, accuracy and reliability become even more critical due to the sheer volume and complexity of the data involved.
Here are four key considerations for ensuring data accuracy and reliability in economic trend prediction:
- Data quality assessment: Conducting thorough data quality assessments helps identify and address any errors, inconsistencies, or biases in the data. This step is essential for ensuring the accuracy and reliability of the predictions.
- Data validation and verification: Employing robust validation and verification techniques can help confirm the accuracy and reliability of the data used for economic trend prediction. This can include cross-referencing the data with multiple sources or conducting statistical analyses to identify any anomalies.
- Data privacy protection: Ensuring data privacy is crucial to maintain the integrity of the data used for economic trend prediction. Implementing appropriate data privacy measures, such as encryption and access controls, helps protect sensitive information from unauthorized access or disclosure.
- Data security measures: Implementing robust data security measures is essential to safeguard the data used for economic trend prediction from cyber threats and unauthorized access. This includes implementing firewalls, intrusion detection systems, and encryption techniques to protect the data throughout its lifecycle.
Real-Time Data Availability
Real-time availability of data is crucial for ensuring the timeliness and accuracy of economic trend predictions. In today’s fast-paced world, where information is constantly changing, real-time data analysis plays a pivotal role in enabling data-driven decision making.
Real-time data analysis refers to the process of analyzing data as it is generated, allowing for immediate insights and informed decision making. This approach enables businesses and policymakers to respond quickly to emerging trends, market shifts, and economic indicators.
By utilizing real-time data analysis, decision makers can make more accurate predictions about future economic trends, identify potential risks, and seize opportunities. Moreover, the availability of real-time data enhances transparency and accountability in economic forecasting, enabling stakeholders to track the reliability and validity of predictions.
Overall, real-time data availability and analysis are essential elements in the quest for accurate economic trend predictions and effective data-driven decision making.
Machine Learning for Economic Forecasting
This discussion focuses on the accuracy of machine learning (ML) models and the quality of data sources. Accuracy is a critical factor in ML models as it determines the reliability of predictions. Data sources and their quality also play a crucial role in ML models, as the accuracy and reliability of the predictions heavily rely on the quality and sufficiency of data.
Furthermore, the impact of ML on decision-making is an important consideration. ML models can influence economic policies and strategies based on the predictions generated by ML models. The accuracy and reliability of these predictions, as well as the quality of the data sources, are crucial factors in determining the impact of ML on decision-making.
In the context of economic forecasting, accurate predictions are essential for making informed decisions. ML models have the potential to improve forecasting accuracy by analyzing large amounts of data and identifying patterns and trends. However, the accuracy of ML models is heavily dependent on the quality and sufficiency of the data sources used.
Accuracy of ML Models
The accuracy of machine learning models in predicting economic trends and market shifts is a crucial aspect of utilizing big data for these purposes. To ensure accurate predictions, proper data preprocessing and model evaluation techniques are essential.
Data preprocessing involves preparing and cleaning the raw data before feeding it into the machine learning models. This step includes handling missing values, outlier detection, and feature scaling. By preprocessing the data, we can eliminate noise and improve the quality of the input, leading to more accurate predictions.
Model evaluation is necessary to assess the performance of the machine learning models. This involves splitting the data into training and testing sets, applying the model to the training data, and then evaluating its performance on the testing data. Common evaluation metrics include accuracy, precision, recall, and F1-score. By evaluating the models, we can identify their strengths and weaknesses, make necessary adjustments, and improve their accuracy in predicting economic trends and market shifts.
Data Sources and Quality
Data sources play a crucial role in generating accurate and reliable predictions in machine learning models for economic analysis. The quality and reliability of the data used for training and testing these models greatly affect their predictive capabilities.
The process of data collection involves gathering relevant information from various sources such as surveys, government databases, financial reports, and social media platforms. Ensuring the accuracy and completeness of the collected data is essential for generating reliable predictions.
Data analysis techniques are then applied to clean and preprocess the data, removing any outliers or errors that may affect the model’s performance. Additionally, data analysis helps in identifying patterns, trends, and relationships within the data, which can provide valuable insights for economic analysis.
Therefore, careful selection and rigorous analysis of data sources are crucial for achieving accurate and reliable predictions in machine learning models for economic analysis.
Impact on Decision-Making
The impact of accurate and reliable predictions in machine learning models for economic analysis on decision-making processes cannot be overstated. These models have the potential to revolutionize the way businesses and policymakers make strategic decisions. However, there are certain challenges that need to be addressed to ensure the ethical and unbiased use of these predictions.
Two key issues that need to be considered are data privacy and algorithm bias.
- Data privacy: As machine learning models require vast amounts of data to make accurate predictions, there is a risk of compromising individuals’ privacy. Strict regulations and safeguards must be implemented to protect sensitive information and ensure that data is anonymized and used responsibly.
- Algorithm bias: Machine learning models are only as good as the data they are trained on. If the training data is biased, the predictions generated by the model will also be biased. Careful attention must be paid to the selection and curation of training data to minimize any unfair biases and ensure that predictions are objective and unbiased.
Challenges and Limitations of Using Big Data in Predicting Market Shifts
Utilizing big data to forecast market shifts faces various challenges and limitations.
One of the main limitations is the quality and reliability of the data used. While big data offers vast amounts of information, the accuracy and relevance of the data can vary significantly.
Additionally, the use of certain data analysis techniques presents limitations. For example, traditional statistical models may not be suitable for analyzing big data due to its volume, velocity, and variety. This necessitates the development and application of new analytical techniques that are specifically designed for big data.
Moreover, the interpretation and understanding of the results obtained from big data analysis can also be challenging. The complexity of big data requires skilled professionals with expertise in data analysis to effectively interpret the findings and make accurate predictions.
Overall, while big data holds immense potential for predicting market shifts, it is important to recognize and address its limitations to ensure reliable and accurate forecasts.
Case Studies: Successful Applications of Big Data in Economic Trend Prediction
Case studies of successful applications in economic trend prediction demonstrate the practical value of incorporating large-scale information analysis. These studies highlight the potential of big data in financial markets and supply chain analysis. Notable examples include:
- Google Flu Trends: Google utilized search data to predict flu outbreaks, providing early warning signals to public health officials.
- Amazon’s Recommendation System: By analyzing customer data, Amazon’s recommendation system accurately predicts consumer preferences, leading to increased sales.
- Walmart’s Supply Chain Optimization: Walmart leverages big data analytics to optimize its supply chain, reducing costs and improving efficiency.
- Airbnb’s Price Prediction: By analyzing various factors, such as location and availability, Airbnb predicts optimal pricing for hosts, maximizing revenue.
These case studies demonstrate the transformative power of big data in economic trend prediction. Incorporating large-scale information analysis can lead to improved decision-making, enhanced efficiency, and increased profitability in various industries.
Ethical Considerations in Using Big Data for Economic Analysis
Ethical considerations surrounding the utilization of large-scale information analysis in economic analysis encompass a wide range of concerns.
One of the primary ethical implications is related to privacy concerns. The use of big data for economic analysis often requires the collection and analysis of a vast amount of personal information. This raises concerns about individuals’ privacy rights and the potential for misuse or unauthorized access to sensitive data.
Additionally, there is a risk of discrimination and bias in the analysis and interpretation of the data, which can have significant ethical implications. The responsibility of ensuring data privacy and mitigating potential biases lies with the organizations and researchers involved in the analysis.
Proper data anonymization techniques, rigorous privacy protections, and transparency in data collection and analysis can help address these ethical concerns and ensure the responsible use of big data in economic analysis.
Frequently Asked Questions
What Are the Potential Ethical Concerns and Risks Associated With Using Big Data for Economic Analysis?
Potential ethical concerns and privacy risks arise when using big data for economic analysis. These include issues of consent, data security, and the potential for discrimination and bias in decision-making processes.
How Reliable and Accurate Are the Predictions Made Using Big Data in Economic Trend Analysis?
The reliability concerns and accuracy limitations of predictions made using big data in economic trend analysis need to be addressed. It is important to evaluate the reliability and accuracy of these predictions in order to make informed decisions.
Are There Any Specific Industries or Sectors Where Big Data Has Been Particularly Successful in Predicting Market Shifts?
Specific industries have experienced successful predictions of market shifts using big data. This suggests that the application of big data analytics has been particularly effective in certain sectors, highlighting its potential in guiding decision-making processes.
What Are Some of the Challenges and Limitations That Arise When Using Big Data for Predicting Market Shifts?
Challenges and limitations arise when using big data for predicting market shifts. These may include data quality issues, limited historical data availability, the need for accurate algorithms, and the potential for biased or incomplete data.
Can Machine Learning Algorithms Effectively Handle and Interpret the Vast Amount of Data Required for Economic Forecasting?
Machine learning algorithms encounter limitations when handling and interpreting the extensive data necessary for economic forecasting. Challenges arise in data interpretation due to its complexity, requiring careful consideration and rigorous analysis.